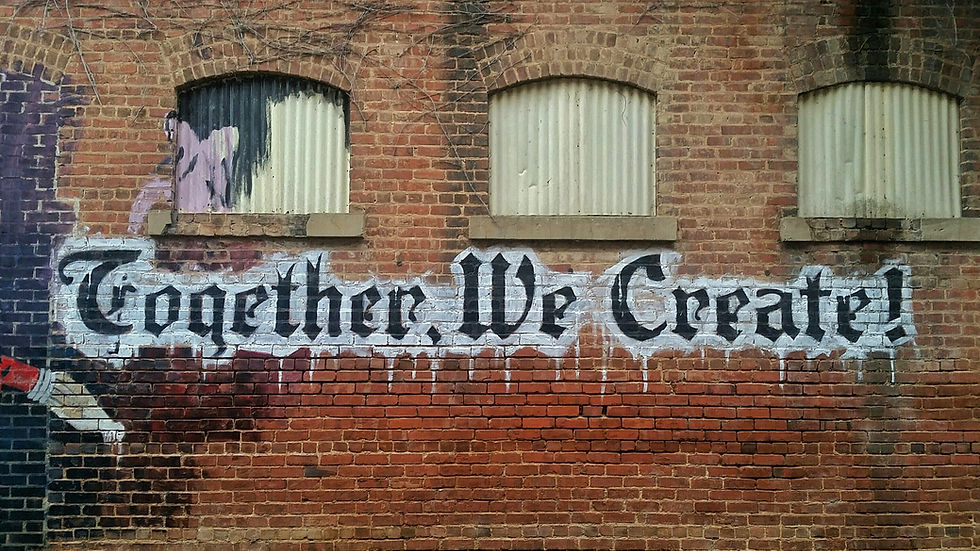
In the dynamic landscape of technology, innovation is the lifeblood of progress. As organizations strive to stay ahead of the curve, generative artificial intelligence (AI) emerges as a powerful catalyst for transformation. In this blog post, we delve into the journey from ideation to pilot implementation, leveraging the capabilities of generative AI.
Understanding Generative AI
At its core, generative AI encompasses machine learning (ML) models trained on massive datasets, enabling them to produce original text, code, images, audio, and video. Large language models (LLMs) and other generative AI techniques drive key advancements in this field.
The Ideation Phase: Seeds of Creativity
Unleashing Creativity with Generative AI Innovation begins with an idea. Generative AI, particularly advanced LLMs, serves as a creativity amplifier. Consider these LLMs:
GPT-3 (and variants): Developed by OpenAI, GPT-3 remains a leader in versatile text generation (Brown et al., 2020).
LaMDA: Google AI's conversational LLM excels at engaging, informative dialogue (Thoppilan et al., 2022).
Gopher: DeepMind's LLM demonstrates impressive abilities to follow instructions (Rae et al., 2021).
These LLMs spark creativity in brainstorming sessions by suggesting unorthodox approaches, exploring untrodden paths, and challenging conventional thinking.
Mapping the Idea Landscape With ideas flowing, generative AI aids in evaluation and prioritization. LLMs analyze data, identify patterns, and predict feasibility. Models like Jurassic-1 Jumbo (AI21 Labs) excel in this type of factual analysis and reasoning. By quantifying risks and rewards, these tools steer decision-making toward impactful initiatives.
The Exploration Phase: Navigating Uncertainty
Prototyping with Generative AI Generative AI bridges the gap between imagination and reality. Code generation models like Codex (Chen et al., 2021) streamline development, while text-based LLMs rapidly draft prototypes, visualizations, and simulations.
Iterative Refinement Generative AI thrives on feedback. As prototypes evolve, feedback from stakeholders guides refinements. The AI system adapts, with recent LLMs like Megatron-Turing NLG (MT-NLG) (Smith et al., 2022) demonstrating remarkable abilities to learn and improve their output.
The Pilot Phase: Taking Flight
From Prototype to Pilot The pilot phase is the runway for innovation. Generative AI assists in transitioning refined prototypes into functional pilots. LLMs can create personalized product recommendations, analyze user behavior, and tailor experiences, all within a controlled pilot environment.
Monitoring and Learning Generative AI tracks performance metrics and adapts to real-world conditions. Continuous learning, especially during the pilot phase, is essential to success. Advanced LLMs continuously fine-tune their behavior, ensuring the project aligns with organizational goals.
Conclusion: The Infinite Horizon
Generative AI is a guiding force in the innovation journey, navigating uncertainty, accelerating prototyping, and transforming ideas into reality. As CIOs and IT leaders embrace these powerful tools, they unlock boundless possibilities across industries and disciplines.
Citations
Brown, T. B., Mann, B., Ryder, N., Subbiah, M., Kaplan, J., et al. (2020). Language models are few-shot learners. Advances in neural information processing systems, 33, 1877-1901.
Chen, M., Tworek, J., Jun, H., Yuan, Q., Pinto, H. P. D. O., ... & Sutskever, I. (2021). Evaluating large language models trained on code. arXiv preprint arXiv:2107.03374.
Rae, J. W., Borgeaud, S., Cai, T., Millican, K., Hoffmann, J., ... & Saeta, T. (2021). Scaling language models: Methods, analysis & insights from training gopher. arXiv preprint arXiv:2112.11446.
Smith, S. L., Kindermans, P. J., Ying, C., & Le, Q. V. (2022). Don't stop pretraining: Adapt language models to domains and tasks. arXiv preprint arXiv:2202.08366.
Thoppilan, R., De Freitas, D., Hall, J., Shazeer, N., Kulshreshtha, A., ... & Le, Q. (2022). LaMDA: Language models for dialog applications. arXiv preprint arXiv:2201.08239.
Important Note: The field of generative AI and LLMs is rapidly evolving. Staying updated on the latest models and research is crucial.
Comments